The Evolution of Video Captioning
Traditional methods like Optical Character Recognition (OCR) and transcription have long served as the backbone of video captioning.
OCR is excellent for extracting text from images and videos, while transcription translates spoken language into written text. However, these methods are limited in scope—they focus solely on either the visual text (OCR) or the audio content (transcription) without considering the full context of a video, such as the relationship between images, sounds, and the underlying scene.
By combining audio, visual, and contextual data into a single, comprehensive caption, this method provides a more holistic understanding of video content.
When integrated with video embeddings and metadata filters, it becomes a powerful tool for hybrid retrieval systems, offering improved precision in Retrieval-Augmented Generation (RAG) workflows.
What Makes Advanced Video Captioning Different?
Unlike OCR, which extracts only the text visible on-screen, or transcription, which captures only the spoken words, advanced video captioning synthesizes information from multiple modalities. It analyzes visual elements, audio cues, and scene context to produce captions that reflect a more nuanced understanding of what is happening in each frame.
For example, in a video where someone is speaking at a podium with applause in the background, traditional transcription might only capture the spoken words, and OCR might miss the context entirely. Advanced video captioning, however, would generate a caption that not only includes the spoken words but also acknowledges the applause and the visual scene of a person at a podium, thereby creating a richer, more meaningful description.
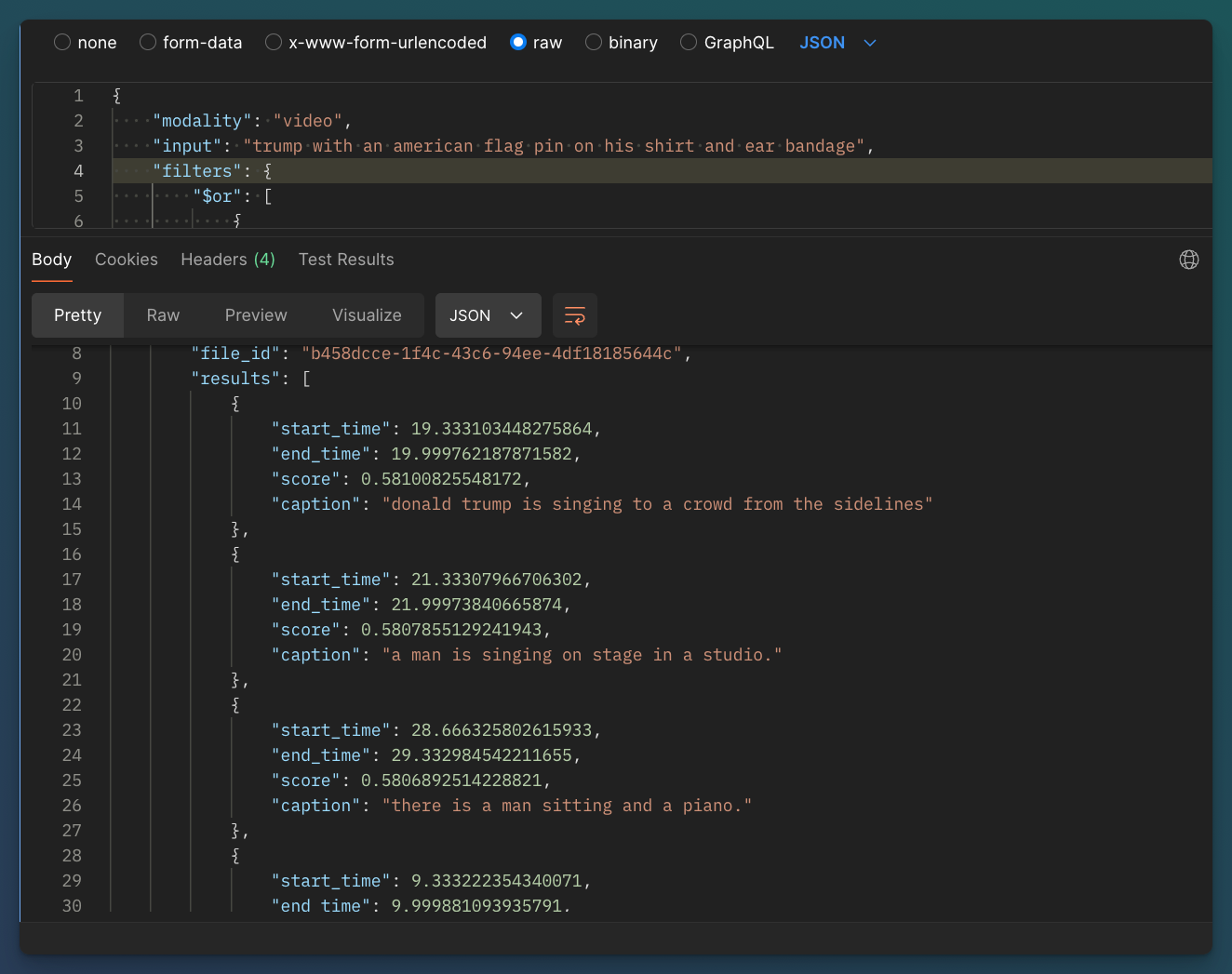
Combining Captions with Embeddings and Metadata Filters for Hybrid Retrieval
Advanced video captioning is particularly powerful when combined with video embeddings and metadata filters in hybrid retrieval systems. Embeddings capture the underlying structure and context of the video content, mapping similar scenes or concepts into a shared vector space. When paired with captions, which provide human-readable descriptions, the system can achieve more accurate and context-aware retrieval.
In RAG workflows, this hybrid approach is invaluable. Metadata filters can further refine the retrieval process by narrowing down search results based on specific attributes like location, time, or speaker identity. The combination of TF-IDF (Term Frequency-Inverse Document Frequency) for captions and KNN (k-Nearest Neighbors) for embeddings enables a robust search mechanism. TF-IDF excels at identifying important terms within the captions, while KNN leverages the embeddings to find conceptually similar content.
Three Use Cases for Combining Captions with Embeddings
- Legal and Compliance Reviews
In legal proceedings, videos often serve as critical evidence. Using advanced captions, legal teams can quickly identify and retrieve relevant clips that contain specific spoken phrases or visual elements, like a person signing a document. Combining captions with embeddings ensures that the search results not only match the keywords but also the context, such as identifying all scenes where the person is interacting with the document, regardless of the exact wording used. - Educational Content Analysis
In educational settings, video content is frequently used to illustrate complex concepts. Teachers and researchers can use advanced video captioning to search for specific moments in a lecture where a particular concept is explained, such as a math problem being solved on a chalkboard. Embeddings help find similar explanations across different videos, while captions ensure that the search results are human-readable and relevant, facilitating more efficient content review and reuse. - Media Monitoring and Content Moderation
In media monitoring, it’s essential to track how certain topics are discussed across various platforms. By combining captions with embeddings, media analysts can quickly find all instances where a specific topic, like a political issue, is mentioned, even if the language used varies. Captions provide clear, human-readable validation, while embeddings capture the broader context, helping to identify related content that might not use the exact keywords.
Fine-Tuning Captions and Embeddings for Specific Use Cases
One of the most significant advantages of this advanced system is its flexibility. Both captions and embeddings can be fine-tuned for specific out-of-distribution use cases. Fine-tuning involves adjusting the model to better recognize and interpret content that it wasn’t initially trained on, making it possible to achieve high precision in specialized domains.
For example, a security firm might need to fine-tune the system to recognize specific actions, such as identifying when someone is retrieving an object from a pocket. While a general model might not excel at this task, fine-tuning the captions and embeddings allows the system to become highly accurate in identifying and contextualizing such actions.
The Future of Video Captioning and Hybrid Retrieval
Advanced video captioning represents a significant leap forward from traditional methods like OCR and transcription. By integrating these captions with embeddings and metadata filters, we can create hybrid retrieval systems that offer unparalleled precision and context-awareness, especially in complex RAG workflows. Whether for legal reviews, educational analysis, or media monitoring, the combination of TF-IDF and KNN in video retrieval provides robust, human-readable results that can be fine-tuned to meet the unique demands of any industry.
As video content continues to grow in volume and importance, the ability to effectively search, understand, and utilize that content will be increasingly vital. With advanced video captioning and hybrid retrieval, we are better equipped than ever to meet this challenge.