This week in multimodal AI:
- Researchers introducing new methods to replace embeddings with discrete IDs for faster cross-modal search
- New video retrieval systems showing improvement by integrating audio with visual cues
- Tutorials to build Multimodal RAG applications
- Multimodal use cases expanding across healthcare and e-commerce
- Cool demos showcasing visual reasoning and multimodal agents
🧠 Research Highlights
- GENIUS – Proposes a universal generative retrieval model that outputs discrete identifiers for targets instead of embeddings (GENIUS: A Generative Framework for Universal Multimodal Search). Significance: Matches the accuracy of embedding-based methods on cross-modal benchmarks with much faster search at scale, pointing to a new efficient paradigm for multimodal retrieval (GENIUS: A Generative Framework for Universal Multimodal Search).
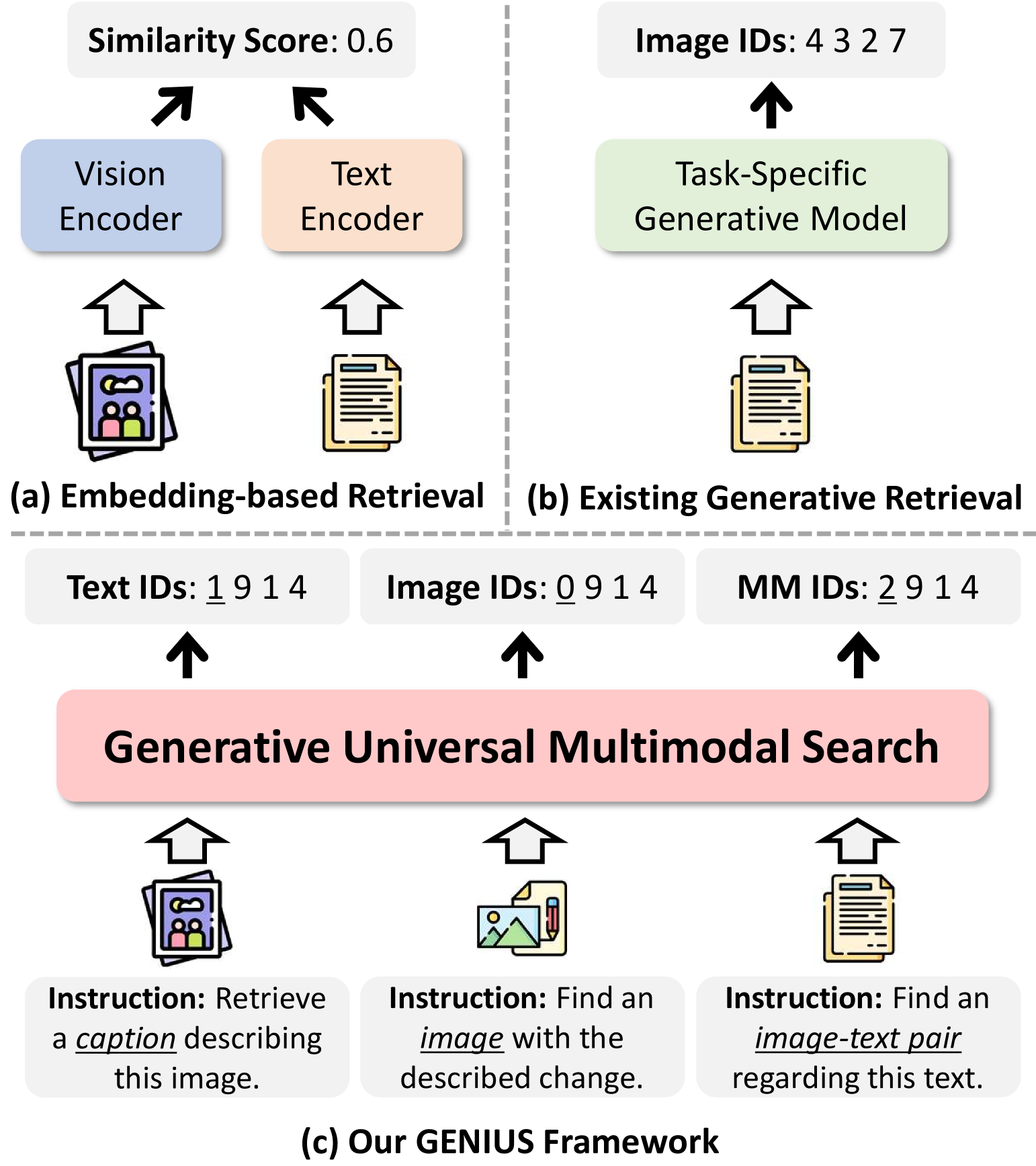
- MegaPairs & BGE-VL – Introduces a synthetic dataset of 26M image-text pairs to train retrieval mod (GitHub - VectorSpaceLab/MegaPairs: MegaPairs: Massive Data Synthesis For Universal Multimodal Retrieval). Significance: The resulting BGE-VL models set a new state-of-the-art on composed image retrieval tasks, outperforming prior methods by ~8% (mAP) and even beating models 50× lar (GitHub - VectorSpaceLab/MegaPairs: MegaPairs: Massive Data Synthesis For Universal Multimodal Retrieval), showing data generation can trump brute-scale.
# Multimodal Retrieval with BGE-VL
# Demonstrates how to use BGE-VL for image + text queries
import torch
from transformers import AutoModel
from PIL import Image
# Load the model - choose from base or large versions
model = AutoModel.from_pretrained("BAAI/BGE-VL-base", trust_remote_code=True)
model.set_processor("BAAI/BGE-VL-base") # Initialize the processor
model.eval() # Set to evaluation mode
# Example: Combined image + text query
# The power of multimodal retrieval is combining both modalities
with torch.no_grad():
# Encode a query using both image and text instruction
query_embedding = model.encode(
images="./product_image.jpg",
text="Find this in blue color with leather material"
)
# Encode candidate images from your database
candidate_embeddings = model.encode(
images=["./candidate1.jpg", "./candidate2.jpg", "./candidate3.jpg"]
)
# Calculate similarity scores
similarity_scores = query_embedding @ candidate_embeddings.T
# Get the most similar item
best_match_idx = torch.argmax(similarity_scores).item()
print(f"Best match: candidate{best_match_idx+1}.jpg with score {similarity_scores[0][best_match_idx]:.4f}")
- MMMORRF – A multilingual video retrieval system that combines visual and audio features using a modality-aware rank fusion (MMMORRF: Multimodal Multilingual MOdularized Reciprocal Rank Fusion). Significance: By integrating multiple modalities, it achieved dramatic gains on video search benchmarks (MMMORRF: Multimodal Multilingual MOdularized Reciprocal Rank Fusion), underscoring the importance of indexing more than just visuals for video understanding.
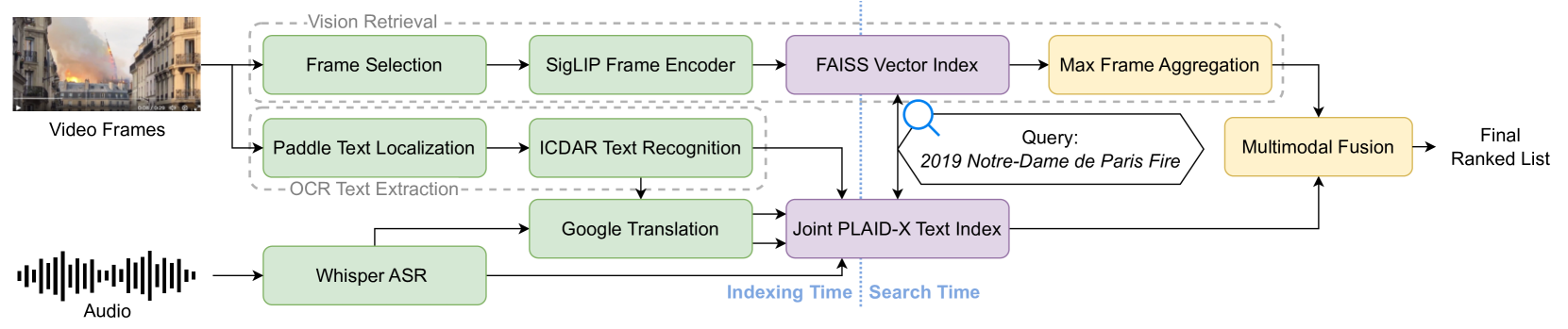
🛠️ Tools & Techniques
- RAP (Retrieval-Augmented Personalization) – A new open-source library (with a CVPR’25 paper) that lets you inject personalized knowledge into a multimodal LLM via retri (GitHub - Hoar012/RAP-MLLM: [CVPR 2025] RAP: Retrieval-Augmented Personalization). How you might use it: You can have a vision-language model “remember” custom concepts (e.g. who your family members are in photos) by feeding it a private image-text database, enabling personalized Q&A or content generation without retraining.

- Multimodal RAG with ImageBind + Elasticsearch – A step-by-step tutorial from Elastic shows how to build a retrieval-augmented generation pipeline unifying text, images, audio, and even depth (Building a Multimodal RAG system with Elasticsearch: The story of Gotham City - Elasticsearch Labs). It uses Meta’s ImageBind to embed different data types into one vector space and Elasticsearch for fast k-NN se (Building a Multimodal RAG system with Elasticsearch: The story of Gotham City - Elasticsearch Labs). How you might use it: Follow their open notebook to spin up a “crime scene AI” that indexes clues of various modalities and lets a GPT-4 agent reason over them to solve myste (Building a Multimodal RAG system with Elasticsearch: The story of Gotham City - Elasticsearch Labs) – a template for any app needing multimodal search with an LLM backend.

🏗️ Real-World Applications
- Healthcare: Google Cloud’s Vertex AI Search for healthcare just went multimodal – doctors can now query patient data with both text and medical images thanks to a new Visual Q&A fe (Google Cloud Enhances Vertex AI Search for Healthcare with Multimodal AI | bakercityherald). This gives clinicians a more comprehensive view (e.g. analyzing X-rays alongside notes), as multimodal analysis of diverse patient data helps improve diagnosis and decision-m (Google Cloud Enhances Vertex AI Search for Healthcare with Multimodal AI | bakercityherald).
- E-commerce: Online retailers are leveraging multimodal AI to enrich product search. For example, Amazon researchers showed that a multimodal LLM can extract product attributes implicitly shown in images or text descrip (EIVEN: Efficient implicit attribute value extraction using multimodal LLM - Amazon Science). This approach (dubbed EIVEN) outperforms traditional methods while using far less labeled data, making product catalogs more searchable and acc (EIVEN: Efficient implicit attribute value extraction using multimodal LLM - Amazon Science) (EIVEN: Efficient implicit attribute value extraction using multimodal LLM - Amazon Science).
- Assistive tech: Multimodal systems are being applied to improve accessibility. Alibaba’s Qwen2.5-Omni, for instance, can generate real-time audio descriptions of visual scenes, which could help visually impaired users navigate their enviro (Alibaba Cloud Releases Qwen2.5-Omni-7B An End-to-end Multimodal AI Model - Alibaba Cloud Community). Similarly, the model can analyze live video (e.g. a cooking demo) and guide a user through tasks step by step, hinting at more intuitive AI assistance in daily (Alibaba Cloud Releases Qwen2.5-Omni-7B An End-to-end Multimodal AI Model - Alibaba Cloud Community).
📈 Trends & Predictions
- “All-in-One” models: There’s a clear movement toward unified models that handle many modalities simultaneously. The debut of Qwen2.5-Omni (vision + audio + text in one) and other efforts (e.g. Google’s Gemini vision-language upgrades) suggest that future AI stacks will favor integrated multimodal understanding over siloed models – all while keeping model size manageable (7B parameters in Qwen’s case, optimized for edge depl (Alibaba Cloud Releases Qwen2.5-Omni-7B An End-to-end Multimodal AI Model - Alibaba Cloud Community).
- Retrieval-augmented multimodality: Many updates this week highlight retrieval as a crucial component of multimodal systems. From using external knowledge to ground image answers, to personalization via retrieved user data (RAP), to generative retrieval replacing indexes – combining search with multimodal models is becoming a standard strategy to boost accuracy and controllability.
- Efficiency and scalability: A growing theme is making multimodal models and searches more efficient. Approaches like GENIUS avoid costly nearest-neighbor search by generating IDs on t (GENIUS: A Generative Framework for Universal Multimodal Search)3-L68】, and new architectures (e.g. Qwen’s blockwise encoders and Thinker-Talker design) enable streaming inputs/outputs without giant compute ov ([2503.20215] Qwen2.5-Omni Technical Report). We foresee a push toward resource-friendly multimodal AI that can run in real-time and at scale.
🧩 Community + Shoutouts
- QVQ-Max visual reasoning demo: The Alibaba AI team unveiled QVQ-Max, a visual reasoning module for their Qwen chat—allowing users to upload an image or video and then see the model’s step-by-step “thinking” process when answering a question (Multimodal: AI News Week Ending 03/28/2025 - Ethan B. Holland). This peek under the hood of a multimodal LLM got the community excited about new ways to interpret and trust AI’s visual answers.
- Together Chat (multimodal agent): Startup Together released a free web demo that combines several open-source models to handle diverse tasks. Dubbed Together Chat, it can perform web search, code writing, image generation, and even image analysis in one in (Multimodal: AI News Week Ending 03/28/2025 - Ethan B. Holland). It showcases the power of connecting multimodal tools (like a language model + vision model) – all accessible to users for free, demonstrating the community’s push toward open, all-in-one AI assistants.